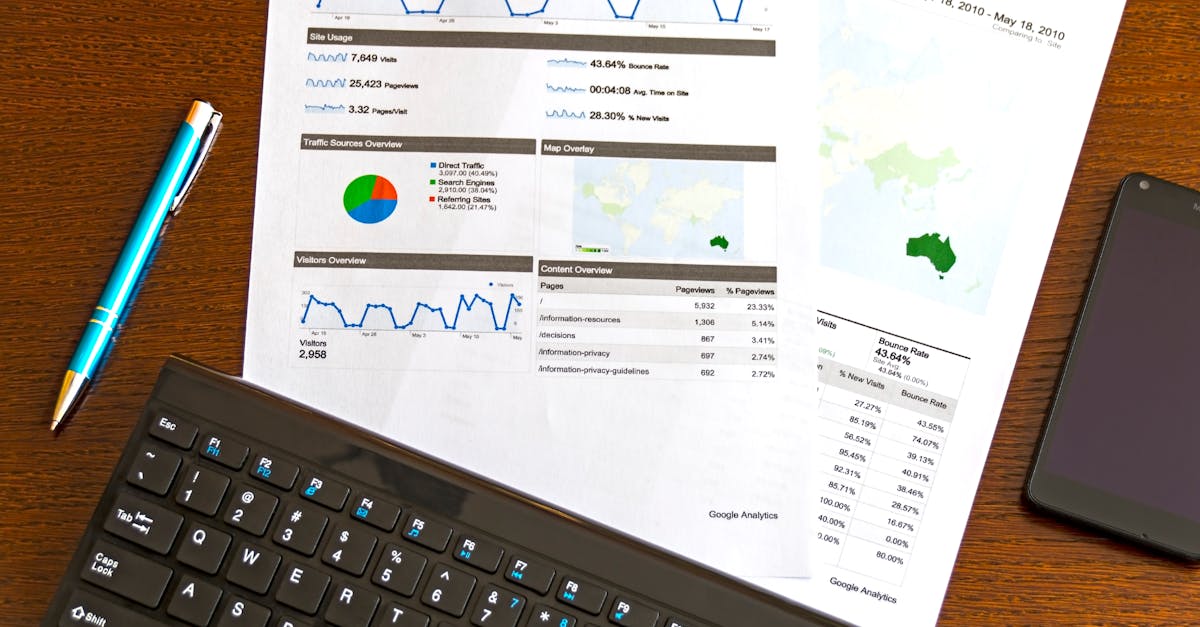
What does SRS mean in statistics?
Standardized residual is the difference between the observed value and the mean value for a population. If an observed value is less than the mean value, it is called a negative residual. If an observed value is greater than the mean value, it is called a positive residual.
A residual is “standardized” if it is divided by the standard deviation for the population. That is, the residuals are divided by the standard error of the population. The purpose of standardizing residuals is to Standardized residual is one of the most popular statistics used in regression analysis and hypothesis testing.
It is a residual that is adjusted for the standard deviation of all the data points that are plotted on a graph. For example, you have collected the heights of 20 people. The average height of these people is 5 feet 6 inches.
If you use the SRS, then you will find the residual of each person’s height when multiplied by the standard deviation of the data. The residuals will take In statistics, the SRS or standardized residual is the difference between the observed value and the mean value for a population. If an observed value is less than the mean value, it is called a negative residual.
If an observed value is greater than the mean value, it is called a positive residual. A residual is “standardized” if it is divided by the standard deviation of the population. That is, the residuals are divided by the standard error of the population.
The purpose of
What does SRS mean in statistics meaning?
Standardized residuals are the differences between observed data values and the expected values under the statistical model. For example, the values collected from the data set about the average length in inches of the population of all the frogs observed in a given area could be used to determine the average length of all the frogs in that area.
Assume that the observed value for the average length of the frogs is 4.76 inches. To find the statistical model that best predicts this value, you could use a regression model Standardized regression score is a measure of how well a statistical model fits the observed data (variance not accounted for by the model).
It is obtained by dividing the residuals of the model by the estimated standard deviation of the residuals. The residual is the difference between the observed value and the estimated value, obtained by the model.
The statistical model is used to explain the relationship between the independent variable (X) and the dependent variable (Y). So, for example, if the dependent variable is the weight of the frogs and the independent variable is the length of the frog, the statistical model can be used to determine the expected weight of a frog based on its length.
If the residuals are plotted against the values of the independent variable, the regression line is drawn.
The residuals are the difference between the observed value of the
What are the original meanings of SRS?
Sample, replication, and sample size maintain their original meanings. The first two are self-explanatory: sample refers to the small segment of data used for analysis, while replication refers to the process of running the same statistical analysis on an entirely new sample of data.
Sample size refers to the number of observations used to calculate a population mean or other measure of central tendency. Analyses can be done on data sets with any sample size, but it’s always best to use the largest sample Short for standardization, residualization, and shrinkage, SRS is a statistical technique that was developed in the 1950s by Ronald Fisher as a way to reduce the effects of outliers in a sample, making the sample more representative of the population.
There are several other statistical techniques that accomplish the same thing, including the Box-Cox transformation and Winsorizing.
When SRS first came into use in the 1950s, it was known as “standardization.” The idea was that when analyzing data, check for patterns of statistical bias and use a “standardization” procedure to make the data more uniform.
“Residualization” refers to removing the effects of the population’s mean from each data point, and “shrinkage” refers to weighting each data point using a factor that maintains the
What do SRS mean in statistics?
Standardized residuals are the differences between observed values and values predicted from a statistical model. They are often referred to as residuals because they are the remaining part of the data after the estimated values have been subtracted from the observed values.
In a regression model, SRS are the difference between each data point and the sample mean. Standardized residual is the difference between the observed value and the expected value. The expected value is a number calculated based on the relevant population using a statistical model.
The mean is the average of all the data in a sample, and the standard deviation is the amount of variation among the sample. A residual is the difference between the observed value and the value expected based on the statistical model. Standardized residual is the difference between the observed value and the value expected based on the statistical model.
A residual is not a number, but a residual value is a variable.
What do SRS mean in programming?
Standardized regression scores (SRS) are statistics that are used to represent the amount of change in a dependent variable that is explained by a predictor when controlling for other independent variables. These statistics are used in predictive models to produce more accurate estimates.
Standardized regression scoring is commonly used in machine learning. It’s a way to normalize the predictions that a machine learning model makes. There are a variety of ways to accomplish standardization, but the simplest approach is to subtract the mean of the output values for each sample and divide by the sample’s standard deviation.
The machine learning community uses the terms standardized regression score and regression score interchangeably. But in the context of software development, a regression score is the output of a regression model that’s been normalized to have a mean of 0 and a standard deviation of 1.
To put it more simply, a regression score is the difference between the actual value of a dependent variable and the model’s prediction of that dependent variable, adjusted for the standard deviation of that dependent variable.